The Concord® team recently sat down with David Meza, the Chief Knowledge Architect at NASA’s Johnson Space Centre. With a background in computer and information sciences, Meza has held various roles at NASA for more than 20 years, from IT management to workspace engineer, overseeing 12,000 computer systems at the Johnson Space Centre (JSC).
Meza said the story of NASA’s bold move into sophisticated knowledge management begins with the simple story of a taxonomist and a web developer. The two met to establish protocols for the release of data to one another, but could not agree on the terms of the exchange. At an alignment meeting, it was later discovered that they were both using the word metadata, but had completely different ideas about what metadata meant. This single meeting triggered Meza’s thinking and eventually led to the creation of NASA’s Knowledge Architecture Office.
In our wide-ranging discussion, Meza touched on six concepts that can help capital project leaders in their own efforts to establish a knowledge architecture.
1| Understand the three components of an effective Knowledge Architecture
When Meza and his team set up NASA’s first Knowledge Architecture Office, it took them several years to identify and fully define the three pillars of a complete Knowledge Architecture: Knowledge Management, Knowledge Sharing and Knowledge Extraction. The catalyst was that fateful miscommunication between the taxonomist and the web developer.
“That was when we first identified the need for a group dedicated to managing all aspects of not only Knowledge Management, but Information Sciences, or Informatics within JSC. In other words, JSC needed a dedicated group that could speak divided languages using the same vocabularies. A dedicated group to provide the liaison needed to make projects work effectively to minimize the likelihood of rework and disputes.
That realization was the foundation of the idea for a Knowledge Architecture Office within NASA. The KAO’s role was to enable the sharing of information and to make it easier for a Knowledge Management Strategy to be implemented on an information science platform. …
A couple of years went by as we first established the role of the KAO. We then realized there was still at least one more aspect missing, related to how we extracted knowledge from our data. What tools, methodologies, and algorithms, were we using to extract the knowledge from our data? Knowledge extraction is when data science came into play, an interdisciplinary field about scientific methods, processes, and systems to extract knowledge or insights from data in various forms, either structured or unstructured, similar to Knowledge Discovery in databases.
JSC’s wealth of archived knowledge required different types of extraction capabilities, such as topic modeling, random forest decision trees, time series analysis, and other appropriate extraction techniques applied to our data, to convert JSC’s information data into actionable knowledge. We developed a framework called Knowledge Architecture, which is a combination of all three of those concepts: (i) Knowledge Management or strategy, (ii) Information Science or informatics, which is the pipeline to transmit the data, and then (iii) Data Science, which provides the algorithms and methods to help us extract the data, and turn it into actionable knowledge. In that sense, the three main functions of the KAO are: Knowledge Management, Knowledge Sharing and then Knowledge Extraction.”
2 | Raise awareness by identifying a “highly visible” first project
Meza said that NASA’s first big challenge was to raise awareness and get buy-in from people inside the organization. They did it by focusing first on a highly visible project that broke down silos and encouraged everyone to get on the same page.
“The number one challenge was to raise awareness and buy-in from the various entities within the organization on the need for knowledge management capabilities. … We looked at some of the more pressing issues around the organization. We identified a highly visible issue that allowed us to develop an appropriate application, platform, and process, that would aid at resolving the issue.
The nature of work within our organization has been silo-driven, meaning that not only do project teams work in separate spaces and integrate only at a later stage but also, many contractors are involved which makes the overall project environment fragmented. Everybody has their own systems for how they store data, including the type of metadata that they do or don’t keep and the way they share information. It’s really a challenge to try to encourage everyone to work on the same page.”
3 | Use Knowledge Informatics to leverage enterprise search
Meza used knowledge informatics – including sentiment analysis, co-word analysis and time series analysis – to make a persuasive outcome-based argument in favor of Knowledge Architecture and Knowledge Management. The benefits of enterprise search won internal converts who extolled the benefits of Knowledge Management to the rest of the organization.
“There is a group at JSC whose role is to debrief the astronaut as they come down from the International Space Station, asking them questions about life on a space station. How was the equipment? How were the computers? How was the food? How was the training? The sleeping arrangements? Different questions about the pros and cons, what they liked and disliked about the equipment, the systems, and everything they utilized on station.
The debriefs have occurred at the end of every mission, for the last 15 years, when the astronauts return, so we have probably 90,000-plus comments in a SQL database. If a team member initiates a query, for instance “Can you tell me what the astronauts thought about the exercise equipment used over the years?” A Human Factors Engineer would have to go into the database and perform a keyword search on the SQL database. Using the search, the Human Factors Engineer would try to find information using the keywords out of these historic comments, read all of the comments, and try to decipher and give their thoughts about what the Astronauts thought about the exercise equipment. When we’re considering 90,000 historic comments over 15 years, that’s a lot of time for these human factors engineers to have to do that work, and to produce a quality analysis.
Human Factors Team within NASA asked if there was a way that we could help them out, to better explore the database and reduce their search time and increase effectiveness. Our method was to first determine a specific categorical information needed by the team, in this case the sentiment around the exercise system, and exported all of the comments that were in the same category, and then we applied sentiment analysis to those comments. Now we can tell, based on what the Astronaut was saying, whether that comment was a positive or negative, based on the context they were talking about, which in this case was exercise equipment.
Then, we could perform co-word analysis, to be able to align the comments in a superior way. Based on the timing of when the comments were made, we could then do a time series analysis, to see if there was a trend going up or down, on the positive or negative aspects of the equipment. We could see how that was trending, over the years.
Needless to say, the human factors team were delighted with the results of the analysis and the time reduction achieved. Consequently, the Human Factors Team has become our greatest advocates in the kind of analytical work we’re doing. Our process works equally well on unstructured, as well as structured data, showing the possibilities of what can be done with a Knowledge Architecture framework.”
4 | Host a structured data alignment workshop as part of the planning initiative
“The planning initiative must consider the various requirements for analysis of the data stored across the Knowledge Management Life Cycle. In the initiation phase of a project, team members must understand that the Knowledge Management Life Cycle will often be different to the individual Project Life Cycle. That means, the data collected in one project may provide knowledge or intelligence that is useful to many other projects within or outside of the agency. Those projects may have already come to the end of their life cycle, or they may be running in parallel to other projects, or they may not yet have commenced.”
According to Meza, foundational questions to ask during the alignment workshop include:
-
What is the best method for storing information?
-
What is the best method for transmitting and sharing the information from an informatics standpoint, to ensure all appropriate people have access to the information?
-
Am I going to use an API, and if so, what are the most appropriate specifications?
-
What type of database if any am I going to use to store the information, and how should the data be structured within that database?
-
How am I going to analyze the data to provide an effective analysis of the intelligence contained within the data?
Additional alignment opportunities exist around issues such as cost, schedule, scope, and operability as well as metadata specifications, security, documentation management, and data management.
“The discussion should include topics such as, what am I going to do for creating, warehousing, analyzing and visualizing this data, across the project life cycle. The proactive discussion at project initiation must allow everybody to get on the same page from the start of the project. We do not want people acting independently and deciding to create their own isolated databases in their own isolated environments, or developing their own non-standard way of handling data, leading to an even deeper fragmentation of our body of knowledge.”
5 | Establish a Master Data Management Plan and Standard Document Specifications
For Meza, the critical success factor in knowledge management is the implementation of standardized protocols around the creation and storage of information.
“When I talk about Knowledge Architecture I discuss my ideas about the information that should be associated with the data and stored to enable ease of use of the data. The critical success factor necessary to implement a Knowledge Architecture framework, that I always mention, is the concept of a Standard Documentation Specification. A Standard Documentation Specification requires a Master Data Management Plan (MDMP).
It is incredibly important to make sure your organization has an MDMP and is able to understand how they’re going to store their data to a Designed Standard Documentation Specification (DSDS). The type of metadata put into that standard documentation specification is critical to the usability and analysis of the data at a later time.”
5 | Consider defining knowledge management standards in RFPs and contracts
“We definitely should be doing more by defining these standards and requirements in our contracts, when we send out RFPs. We should detail our expectation in terms of data storage. But that’s a challenge for today and for the future. As we’ve become more electronic, it’s just now coming to the forefront that we need to do a better job of how we create and store data, and it needs to be incorporated into our procurement contracts. The issue with that now becomes a legal matter. Now this may be interpreted as a deliverable, leading to an extra cost. There is a trade-off between what we can afford and what we can mandate that needs to be carefully determined. The size of projects varying from very large million-dollar contracts, to very small $200,000 or less contracts also adds another layer of complexity.”
6 | Always begin by defining the starting question and data-driven visualization
C0ncord asked Meza how he organizes and scopes the ontologies that NASA uses in its Knowledge Management protocols. He answered that his first step is always to define the starting question.
“The most important part for our approach is to define the starting question, as initiated by the expert team. I don’t try to apply a scatter approach. I want to understand the questions that are being asked and the answers they are looking for, the type of data they have to search, and then figure out the best way to visualize that information. It’s what I call data-driven visualization. I allow the data to drive how I’m going to visualize it, based on the questions that are being asked or the answers that are being sought from that data. …
Eventually, as we do this more and more, we will come up with a set of processes, workflows, as well as algorithms and methodologies that go with them, that users will be able to utilize to fit their data. As always, the methods will have to be evaluated and refined over time to make sure they’re working properly.”
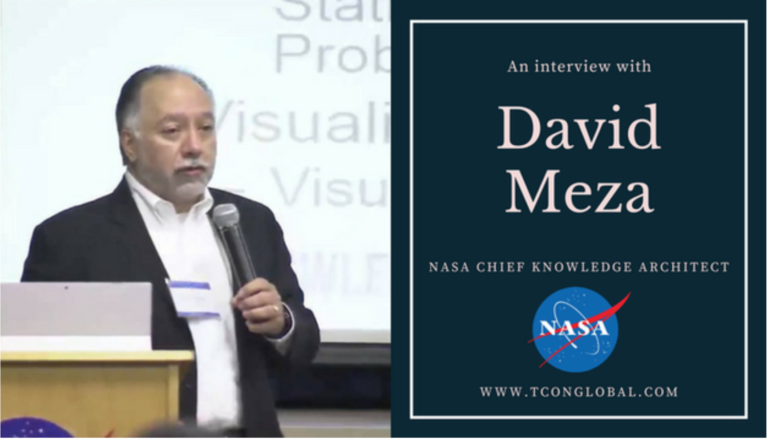